Oh No, Data Strategies: For Data-Driven Enterprises
Introduction
In many of my previous writings, I've written about identity resolution, the never-ending debate on omnichannel vs. multi-channel personalization, and provided a checklist for evaluating Customer Data Platforms (CDP).
Often, my focus, much like many of my peers, has leaned towards the technology side of things. However, technology, no matter how advanced, is merely a tool. The real power lies in the strategies that drive these technological choices, particularly the DATA STRATEGIES that surface use cases. These use cases supposedly drive the technology vendors to create solutions to meet the use case need and ultimately satisfy the strategy's management objectives of growth, revenue, and cost reduction.
Across many organizations, many departments create strategies in silos, each catering to specific mandates and often with competing objectives. While potent in their own right, these strategies rarely converge, leading to fragmented insights and duplicated efforts. The buzz around unifying these strategies into a cohesive, data-driven narrative has existed for a while. For over two-plus decades, we've been bombarded with terms like "data-driven," "digital transformation," and "omnichannel." Giants like Adobe, Microsoft, Oracle, IBM, Google, AWS, and Salesforce have only added to the cacophony, introducing their brand of buzzwords like CX, DX, CDP, DMP, Serverless, Microservices, Modern Data Stack, and Event-driven, often blurring the lines between genuine value and marketing fluff. Candidly, much of it feels like an orchestrated ruse to keep the wheels of the tech and VC industry churning.
This myriad of technologies, now dubbed the "Modern Data Stack," is this new stack metaphor genuinely serving businesses, or is it just an elaborate maze, diverting us from the core objective – harnessing data for tangible growth? While some tools in this stack bring value, others seem like overengineered solutions for straightforward problems. The term has been used widely in the data community to refer to the combination of modern tools and technologies for data collection, storage, processing, and visualization. It encompasses tools like Fivetran, dbt, Snowflake, and Looker. Some of these tools may add unnecessary complexity and proliferate redundant data. Many do not even work well or cause the users to throw up their hands in disgust. While others are terrific, save countless hours and frustration, and provide exceptional value to the organizational use cases that drive their feature sets.
While I've spent significant time on the vendor side, championing newer technologies, I often wonder if organizations, irrespective of their scale, had rigorously implemented and adhered to data strategies from the get-go, would we be navigating this labyrinthine tech landscape today? There's no denying the need for innovation and tool evolution, but there's a fine line between advancement and overcomplication.
Several voices in the industry, many of whom have battled in the trenches of data strategy execution, offer profound insights into this dilemma. Their perspectives, stemming from ground realities, often contrast with mine, shaped by the tech vendor's lens. This dichotomy, in many ways, encapsulates the broader sentiment. e.g., everyone has an opinion. While legacy systems sometimes become tech debt, necessitating newer solutions, does it justify the creation of tech stacks that resemble flashy, over-customized cars roaring down highways? These "hobbyist tech stacks," though fascinating, might not be what businesses genuinely need. The strategies below do not dive deep into specialized tools required for specific tasks but discuss the broader foundational aspect. Organizations should start with buying foundational tools that get them 50-75% of the way there vs. buying hundreds of more flashy add-on tools.
A great site and new book by a LinkedIn connection of mine, Arpit Choudhury - "The Good Data Growth Model" - " and, DataBeats, discusses many data growth concepts that complement my article on the strategies you must deploy. Thank you, Arpit, for letting me reference your great work and illustration below. Arpit's approach goes into much more detail. He illustrates great visuals on how the data flows, as seen below in the image, and how it is the tool to help execute the strategies I propose in this article. I aim to shift the lens from the sparkle of the new modern data stack(technology focus), go above the fold from what Arpit explores in his new book, and create a unifying strategy approach for the varying business teams so they can drive toward Arpit's "Good Data Growth Model - GDGM". The unified strategy lays the roadmap, while Arpit's model is the execution and action. When the individual strategies are unified, we'll explore how those individual data strategies can become a force multiplier for organizations and how GDGM can act as an accelerator. I will start with a journey spanning the foundational pillars of data strategy to a unifying vision for a Center of Excellence model where the GDGM is used by the CoE and applied across the groups. I hope to offer a holistic view, merging business and IT and, hopefully, steering the discourse from tools to tactics, from silos to strategy.
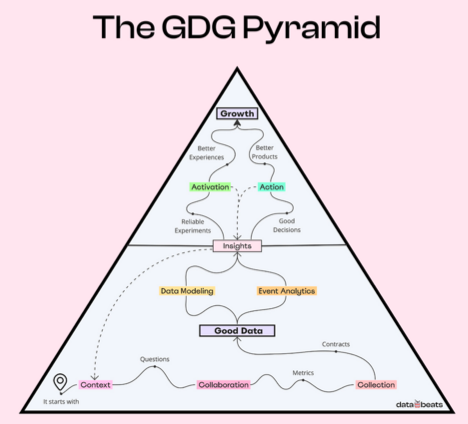
Setting the Stage: The Four Pillars of Data Strategy
Let's get started. In the world of business data management, four primary strategies have emerged as cornerstones in various organizations, each serving unique yet crucial roles:
- Enterprise Data Strategy: Often perceived as the backbone, this strategy focuses on managing the organization's overall data resources. From governance and architecture to quality and security, it ensures data is reliable, accessible, and primed for decision-making.
- Customer Data Strategy: As businesses become more customer-centric, understanding the audience is paramount. This strategy zeroes in on collecting, managing, and analyzing data related to customers, offering insights into their behaviors, preferences, and journeys. Many new terms and technologies are associated with this strategy, making this area all the more confusing to businesses.
- Marketing Data Strategy: Every marketer knows the value of targeted efforts. With the plethora of data available from campaigns, websites, and customer interactions, this strategy aims to optimize marketing efforts. It's about reaching the right audience with the right message at the right time. Most of us have used dozens of different tools promising an array of advances each year, striving to address the Omnichannel promises made over the last 25 years.
- Omnichannel Personalization Strategy: While this has been the promise for the last 25 years, rarely does any company or marketing/sales department, agency, or vendor ever realize it. The promise is the modern customer interacts with businesses across multiple touchpoints – online, offline, social media, and more. This strategy is geared towards offering a consistent, personalized, coordinated experience, no matter where the interaction takes place. While the strategy is discussed and unified below, be aware it is still challenging to achieve and realize. I hope that Gen-AI, combined with newer ML processes and a unified profile, will help in this regard more quickly in the next few years.
While each strategy holds its merits, operating in isolation can lead to fragmented insights, missed opportunities, and redundancies. Imagine the marketing team targeting a segment without insights from the customer data or the IT team focusing on data security without understanding the nuances of customer interactions. These silos hinder an organization's holistic growth and optimization when strategies interweave.
It's this very gap that necessitates a unified approach. Before we dive into the intricacies of such a unified framework, we must appreciate these individual strategies' nuances and understand the inherent challenges in their isolated operation.
Component | Enterprise Data Strategy | Customer Data Strategy | Marketing Data Strategy | Omnichannel Personalization Strategy |
---|---|---|---|---|
Primary Focus | Whole organization's data management | Customer-centric data | Data supporting marketing efforts | Personalized experience across all touchpoints |
Key Objective | Reliable, accessible, and usable data | Deep understanding & improved customer experience | Optimize marketing efforts | Consistent & tailored customer experience across channels |
Value Proposition | Support business decisions & operational efficiency | Gain insights into customer behaviors & preferences | Measure ROI, target audiences effectively | Enhance engagement & loyalty through personalized interactions |
Technological Integration | Data governance tools, Data architecture Infrastructure | CDP, CRM systems, Predictive analytics tools | Campaign management platforms, Audience targeting tools | Unified customer profile platforms, Channel integration tools |
Data Management Focus | Governance, Quality, Security | Collection, Segmentation, Privacy | Campaign data, Audience data, Content personalization | Unified view, Channel data, Real-time engagement, Feedback loop |
Analytical Component | Data architecture, Data integration | Customer profiling, Journey mapping | Marketing ROI analysis, Multi-channel analytics | Real-time analytics, Personalization algorithms |
Integration with Snowflake / Databricks | Data warehousing, Analytics | Data aggregation, Real-time streaming | Data-driven insights, ML algorithms | Real-time data sharing, Streaming analytics |
Third-Party Integration | Data cataloging tools, Security tools | CDPs (e.g. Adobe AEP, Salesforce Data Cloud, Rudderstack, Amperity) | Marketing apps (e.g., HubSpot, Google Analytics) | Omnichannel apps (e.g., Salesforce Commerce Cloud, Braze) |
The Vision: Unified Data-Driven Center of Excellence (CoE) Strategy
Amid the myriad data processes and strategies at play, there's a dire need for an overarching vision that harmoniously interlinks each distinct data strategy, creating a symphony of data-driven actions that propel the organization forward. Enter the concept of a Center of Excellence (CoE).
The Concept of a CoE
At its core, a Center of Excellence isn't just about consolidation. It's about excellence, best practices, innovation, and continuous improvement. A CoE serves as a centralized hub for data management, pooling resources, skills, and technologies to drive strategic and operational decisions.
The Case for a Unified Strategy
The power of the CoE strategy stems from its unified nature. By consolidating the four primary data strategies into one coherent framework, we're not merely combining them but enhancing their cumulative value. Think of it as a relay race where each strategy is an athlete. Rather than running separate races, they're passing the baton to one another, each leveraging the momentum of the other, driving towards a common finish line: organizational success.
Business and IT Alignment
Historically, a chasm has existed between business and IT stakeholders. While business teams focus on growth, market share, and customer satisfaction, IT teams delve into systems, integrations, and data security. The CoE serves as a bridge. It aligns goals, ensures seamless communication, and fosters collaboration, ensuring data becomes the common language spoken organization-wide.
As we further dissect this unified strategy, it will become evident that the CoE is more than a structural change. It's a cultural shift, a reimagining of how data is perceived, managed, and leveraged. It's the lighthouse guiding organizations amidst the vast ocean of data, ensuring every stakeholder, whether in business or IT, sails harmoniously towards shared horizons.
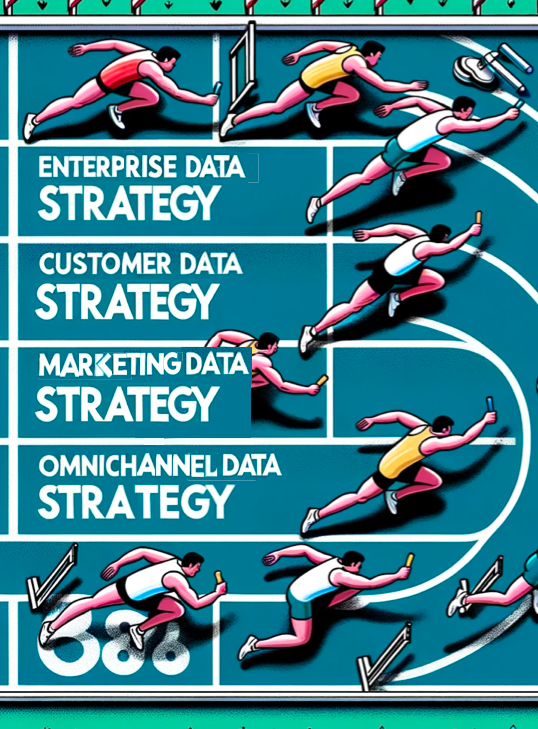
Diving Deeper: Components of the CoE Strategy
The transformative potential of a Unified Data-Driven Center of Excellence (CoE) Strategy lies in its intricate components, each serving a pivotal role in bridging the gaps between business and IT. There are many tools and vendors in this genre. Many have multiple components that will satisfy this area, like data catalogs, Data discovery, metadata repos, AI/ML, Data lakes, Lake houses, ETL, ELT, et al... I mention a few below, but there are many different layers and directions I could elaborate on. I will focus mostly on Snowflake and Databricks as foundational technologies, and I name a few others as complements or augmentations to those two platforms. Note that the list is not exhaustive and only illustrative. Snowflake and Databricks, as mentioned below, could handle 75-90% of the components named, but often there are better specialty products that will be better in a more composable manner versus buying the complete suite of the two foundational platforms. However, an enterprise could opt-in to buying the entire Snowflake or Databricks suite of tools. Here is a site that helps with these areas in more depth: The NewStack. Let's dissect a few of these components, understanding their essence, interplay with other tools, and ultimate value to the organization. I have a bit of a checklist here if you are interested in more depth.
Integrated Data Governance & Management
This is the foundation upon which all other components are built. It's about ensuring that data, the most valuable asset of a modern organization, is managed with the utmost precision and care.
- Tools & Technologies: Leveraging platforms like Collibra, Apache Atlas or Alation for governance, data catalog, data discovery, data lineage, or ensuring data quality to standardize organizational practices.
- Value Proposition: By ensuring data quality and security, businesses can rely on their data for decision-making, reducing risks associated with inaccuracies or breaches.
- Snowflake/Databricks Role: I picked these two as they are the most predominant players for the enterprise data architecture platforms. Snowflake's secure data sharing combined with Databricks' Delta Lake ensures data reliability and streamlined governance. This idea and approach may seem contradictory, but there are many instances where the two are complementary vs competitive. However, this line is narrowing daily as each vendor stakes more claims into each other's territories. Also, both are going more horizontal, left and right. To the left into the cloud infrastructure space and to the right into the Marketing, Sales, ERP, and Service data apps.
Unified Customer 360 View
In a world where customer experience can set companies apart, having a comprehensive understanding of every customer is invaluable.
- Tools & Technologies: CDPs and CRM systems like Adobe, Treasure Data, Simon Data, and Salesforce can be augmented with integration platforms like Coalesce, Workato, Keboola, Estuary, Snaplogic, and Nexla to pull data from any touchpoints. For Identity Resolution, you might incorporate Zingg.ai or Amperity.
- Value Proposition: A 360° view enhances personalization capabilities, improving customer satisfaction and potential upselling opportunities.
- Snowflake/Databricks Role: With Snowflake acting as a centralized data hub and Databricks processing diverse data sources, creating a holistic customer view becomes seamless.
Collaborative Analytics & Insights
Data's actual value is unlocked when interpreted and acted upon. Collaborative analytics ensures that insights are not siloed but shared, refined, and acted upon collectively.
- Tools & Technologies: Utilizing Business Intelligence tools like Tableau can democratize data insights, while platforms like Microsoft's new Fabric Analytics foster collaboration. There are many in this space, too many to list.
- Value Proposition: Shared insights lead to unified, informed decisions, ensuring different departments move cohesively towards organizational goals.
- Snowflake/Databricks Role: Snowflake's data-sharing capabilities, combined with Databricks' collaborative Data science and analytics notebooks, can enable real-time insights and collaborative analytics.
Data-driven Marketing & Omnichannel Integration
Today's customers interact with brands across a multitude of channels. Ensuring consistency and personalization across these touchpoints is paramount. I have written a bit about this topic and plan to write more. Check out the other articles I have written.
- Tools & Technologies: Platforms like Adobe Experience Cloud offer omnichannel solutions, while tools like Braze enhance customer engagement.
- Value Proposition: Consistent, personalized experiences boost brand loyalty and customer lifetime value.
- Snowflake/Databricks Role: By integrating real-time data across channels using both Snowflake and Databricks, marketers can tailor experiences on the fly, reacting to customer behaviors instantaneously.
Continuous Learning & Adaptation
The digital landscape is ever-evolving. For a strategy to remain effective, it must adapt, learn, and iterate.
- Tools & Technologies: Feedback platforms like Qualtrics can provide invaluable insights, while A/B testing tools like Optimizely can validate strategic tweaks.
- Value Proposition: By continuously refining the strategy, organizations remain agile, relevant, and ahead of the curve.
- Snowflake/Databricks Role: Both platforms, through their analysis capabilities, can identify areas of improvement, feeding into the iterative refinement process.
By understanding these components in-depth, stakeholders from the business and IT realms can appreciate the CoE strategy's holistic approach. It's a synergy of tools, data, and collaborative efforts, driving towards a singular goal: organizational excellence through data.
Component | Description & Purpose | Tools & Technologies | Value to Organization | Snowflake/Databricks Integration |
---|---|---|---|---|
Unified Data Governance | Establish standards for data quality, security, and compliance to serve the needs of the entire organization. | Data governance tools like Collibra, Alation. Data quality tools like Talend, Trifacta. Security tools like Varonis, Vormetric. | Ensures consistency, reliability, and security of data across all departments. | Snowflake's secure data sharing, Databricks' Delta Lake for data reliability. |
360° Customer View | Aggregate and consolidate customer data from all touchpoints for a complete understanding of customer behavior and preferences. | CDP and CRM systems like Adobe, Salesforce, HubSpot. Data integration platforms like Keboola, Estuary,or Workato. | Enables a deeper understanding of customers to enhance personalization and service. | Snowflake's single, integrated platform for all data, Databricks for data processing. |
Collaborative Analytics | Foster inter-departmental collaboration on data analysis to drive unified, informed decisions. | Business Intelligence tools like Tableau, Looker. Collaboration platforms like Microsoft Fabric and Teams, Salesforce Slack. | Enhances decision-making through combined insights and reduces duplication of efforts. | Snowflake's seamless data sharing capabilities, Databricks' collaborative notebooks. |
Omnichannel Integration | Ensure a consistent and personalized customer experience across all touchpoints. | Omnichannel platforms like Adobe Experience Cloud, Pega Systems Customer engagement tools like Braze, Demandbase. | Boosts customer loyalty and satisfaction by delivering consistent and personalized interactions. | Snowflake & Databricks for real-time data integration and analysis across channels. |
Continuous Learning & Iteration | Implement feedback loops for continuous refinement of data strategies. | Feedback platforms like Qualtrics, SurveyMonkey. A/B testing tools like Optimizely, Adobe Target. | Ensures the strategy remains up-to-date, effective, and aligned with organizational goals. | Snowflake & Databricks for real-time feedback analysis and strategy refinement. |
Technological Scalability | Ensure the tech infrastructure supports the growth and evolution of the unified data strategy. | Cloud platforms like AWS, Azure. Scalability tools like Kubernetes, Docker. | Provides flexibility and agility to adapt to evolving business needs without major overhauls. | Snowflake's cloud-native architecture, Databricks' scalable Apache Spark integration. |
Stakeholder Training & Development | Equip all stakeholders with the skills and knowledge to leverage the CoE's offerings effectively. | E-learning platforms like Coursera, Udemy. In-house training sessions. | Enhances the effectiveness of the CoE by ensuring all stakeholders are aligned and competent in its utilization. | Snowflake & Databricks webinars, workshops, and training modules. |
This table provides a comprehensive overview of the Unified Data-Driven CoE Strategy. It emphasizes the role of Snowflake and Databricks as the foundational hub for the strategy, ensuring stakeholders understand their centrality in driving this unified approach.
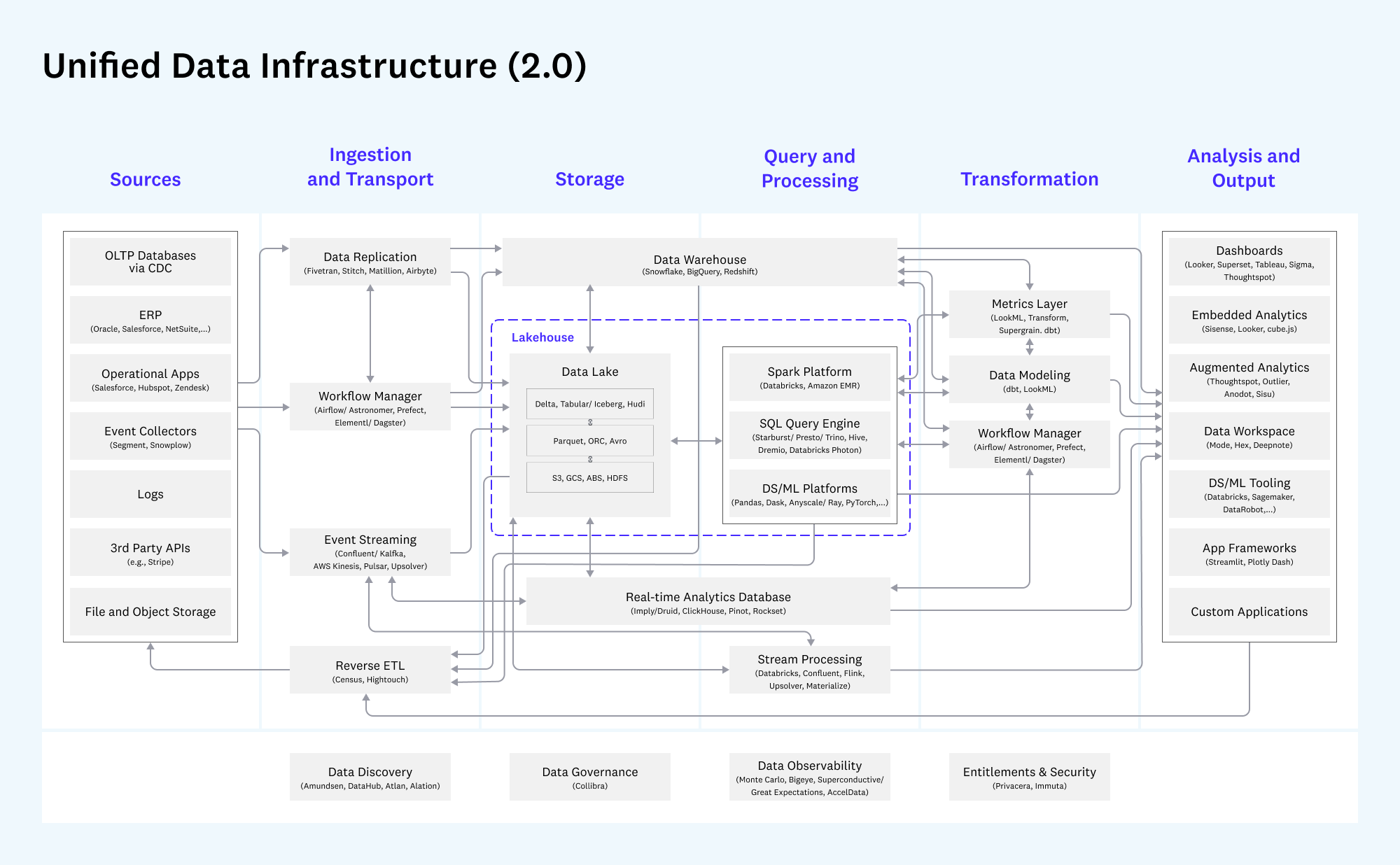
I personally do not believe we need everything here for every organization, but it is a good reference to benchmark against. I also do not agree with every tool they depict. However, they combine Snowflake and Databricks, as illustrated in the article. There are instances where this could be useful and needed based on the data situation. In most cases, it is probably overkill for more organizations, as there is much overlap between the two platforms.
Use Cases: Tactical Implementation
While understanding the components of a Unified Data-Driven Center of Excellence (CoE) Strategy is crucial, its true essence comes to life when we witness its tactical implementation. Real-world use cases offer tangible insights into how this strategy can be executed, addressing the diverse needs of business and IT stakeholders while driving real value for the organization.
Data Cleansing Workshop for Governance
- Execution: Organize regular workshops, bringing IT specialists and data stewards from various departments. These sessions focus on identifying inconsistent, outdated, or redundant data and rectifying them using standardized procedures.
- Value Proposition: With cleaner data, reporting accuracy improves, leading to better-informed strategic decisions.
- Snowflake/Databricks Role: Using Snowflake's data warehousing capabilities alongside Databricks' processing prowess, these workshops can swiftly identify discrepancies and streamline the cleansing process.
Customer Retention Analysis
- Execution: Harness the power of the unified customer view to analyze interaction patterns and purchase histories regularly. Identify potential at-risk customers and proactively engage with personalized retention strategies.
- Value Proposition: Early identification of churn allows businesses to retain valuable customers, maintaining revenue streams.
- Snowflake/Databricks Role: With customer data aggregated and processed in Snowflake and Databricks, predictive models can be employed to forecast churn and devise retention strategies.
Monthly Data Insight Sharing Sessions
- Execution: Facilitate sessions where different departments present vital data insights, fostering a culture of knowledge sharing and collaboration.
- Value Proposition: Encourages cross-departmental understanding of trends, challenges, and opportunities, paving the way for cohesive decision-making.
- Snowflake/Databricks Role: Snowflake's seamless data-sharing capabilities, combined with Databricks' collaborative notebooks, provide the platform for these insights to be collated, analyzed, and shared in real time. While both may be able to provide these tools in isolation, they might have a more significant benefit together. Both have extensive integration into other visualization tools that may provide deeper corporate transparency.
Personalized Omnichannel Marketing Campaigns:
- Execution: Use the unified customer data to drive personalized marketing campaigns across all channels, ensuring customers receive targeted messages based on their behaviors and preferences.
- Value Proposition: Tailored campaigns resonate better, leading to higher engagement rates and better marketing ROI.
- Snowflake/Databricks Role: By integrating data across channels in real-time using Snowflake and Databricks, marketing teams can ensure that the most recent customer interactions inform campaigns.
Quarterly Feedback Loop Integration
- Execution: Implement a system where feedback from all departments is regularly collected, analyzed, and used to refine the overarching data strategy.
- Value Proposition: This iterative approach ensures the strategy remains adaptive, relevant, and aligned with evolving organizational goals.
- Snowflake/Databricks Role: Feedback data stored and analyzed in Snowflake and Databricks allows for swift strategy adjustments based on real-time insights.
These use cases offer a glimpse into the tactical possibilities of the CoE strategy. By aligning tools, processes, and stakeholder objectives, they showcase the strategy's capability to drive organizational growth, efficiency, and adaptability.
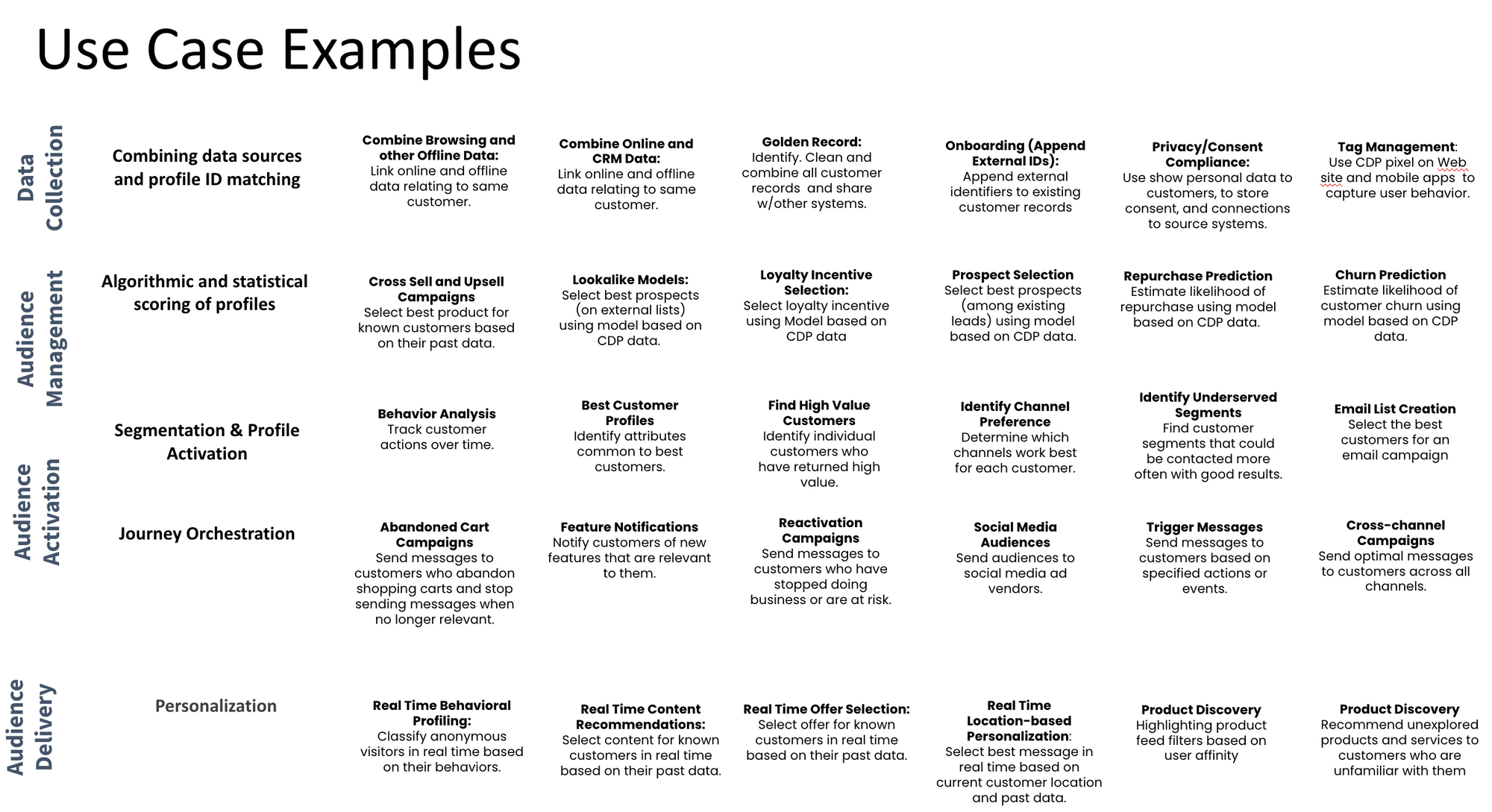
Bridging the Gap: Collaborative Efforts in Action
Beyond the technical intricacies and strategic components lies the human element, often the linchpin of any successful organizational strategy. Bridging the often-existing gap between business and IT stakeholders is fundamental to the success of a Unified Data-Driven Center of Excellence (CoE) Strategy. Let's explore how collaborative efforts play out in real-world contexts.
Cross-functional Teams for Unified Goals:
- Execution: Form teams composed of members from marketing, sales, IT, data analytics, and other relevant departments. These teams collaboratively define objectives, share insights, and drive projects with a unified perspective.
- Value Proposition: Combining expertise from diverse areas ensures well-rounded project outcomes and avoids the tunnel vision that can arise from siloed departments.
- Snowflake/Databricks Role: With collaborative features in Databricks, such as shared notebooks, and Snowflake's capability to grant varying data access levels, cross-functional teams can work cohesively on shared platforms.
Regular Strategy Alignment Workshops
- Execution: Organize monthly or quarterly workshops where business and IT stakeholders review the ongoing data strategy, ensuring alignment with business objectives and technical feasibility.
- Value Proposition: Ensures the strategy remains relevant and adaptive and caters to the organization's evolving needs.
- Snowflake/Databricks Role: Utilize Snowflake and Databricks' analytics capabilities to showcase real-time performance metrics, trends, and areas of improvement during these workshops.
Collaborative Training Sessions
- Execution: Curate sessions where IT teams explain new technologies, data tools, or methodologies to business stakeholders. Conversely, business teams can share market trends, customer insights, and business challenges.
- Value Proposition: Mutual understanding fosters respect, smoother communication, and a more harmonious working environment, leading to better project outcomes.
- Snowflake/Databricks Role: Leveraging Snowflake & Databricks training modules and webinars to ensure technical and non-technical teams are on the same page regarding platform capabilities.
Feedback and Iteration Loops
- Execution: Establish a system where business and IT stakeholders can provide feedback on ongoing projects, tools, or strategies. Use feedback to iterate and refine approaches continuously.
- Value Proposition: Encourages a culture of continuous improvement and addresses challenges or inefficiencies swiftly.
- Snowflake/Databricks Role: Analyzing feedback data in Snowflake and Databricks can highlight patterns or recurring issues, informing the iteration process.
Joint Pilot Projects
- Execution: Before rolling out large-scale initiatives, run pilot projects with a mix of business and IT stakeholders. The pilots ensure feasibility checks, alignment with business goals, and technical optimization.
- Value Proposition: Reduces the risk of project failures, wasted resources, or misalignments between business objectives and technical implementations.
- Snowflake/Databricks Role: Utilize Snowflake and Databricks to quickly prototype, test, and analyze pilot project results, ensuring data-driven decision-making. Both vendors have layers of free capabilities to test ideas depending on your relationship with them.
By focusing on collaborative efforts and bridging the gap between business and IT, organizations can unlock the true potential of a Unified Data-Driven CoE Strategy. It's not just about the data or the tools but about the people driving these initiatives, their expertise, and their collaborative spirit.
Getting Started: Steps for Implementation
Establishing a Unified Data-Driven Center of Excellence (CoE) Strategy can seem daunting. Still, with a well-charted course, the transformation becomes manageable and invigorating for the team involved. As we venture into the steps for implementation, it's crucial to approach this not as a mere series of tasks but as a holistic evolution, engaging every echelon of the organization.
Understanding the Current Landscape
Before any meaningful change can occur, the CoE Group must comprehensively understand the present state of each department across the organization and its requirements for data use. This process involves in-depth audits of existing data practices, tools, systems, and current strategies. Conversations with stakeholders from various departments can shed light on current challenges, aspirations, and perceived gaps in the current data strategy. Assessing the current landscape also involves evaluating the technical prowess of your teams and the state of your data - its cleanliness, consistency, structure, and the technology stacks currently in play. Contracting a 3rd party for this scope of work often benefits deeper insights and analysis. They can also act as mediators because internal ownership and politics can impede collecting all the correct data to make the proper assessments later on when the CoE makes decisions.
Formulating the Vision with Stakeholder Buy-in
A CoE isn't just a technical endeavor; it's a vision. This vision needs to be co-created with inputs from executives, business stakeholders, and IT/DEV teams. Workshops or brainstorming sessions can be organized where stakeholders express their expectations and concerns. The buy-in from leadership is paramount here. When the top echelons champion the cause, it creates a ripple effect, ensuring smoother adoption.
Customized Roadmapping
While the CoE strategy provides a framework, its implementation needs to be tailored to the specific nuances of each organization. It involves prioritizing components based on current needs and foreseeable future challenges. Given its current state, your organization must prioritize data governance before delving into omnichannel strategies. This phase involves creating a detailed, phased roadmap with milestones, KPIs, and clear ownership of tasks.
Integration of Key Technologies
With Snowflake and Databricks acting as the foundational hubs, the integration phase ensures these platforms communicate seamlessly with existing systems and new tools outlined in the strategy. The beauty of both Snowflake and Databricks lies in their ability to integrate with a wide range of tools, making them invaluable assets in this transformative journey. Detailed workshops, led by technical teams, can guide departments on leveraging these platforms to their fullest potential.
Continuous Training and Knowledge Transfer
As the strategy unfolds, continuous training sessions become crucial. It isn't just about understanding the tools but consuming the ethos of a data-driven culture. Customized training modules, depending on the audience – executives, marketers, or IT specialists – ensure that the entire organization marches in lockstep. Regular knowledge transfer sessions, where teams share their successes, challenges, and learnings, foster an environment of collective growth.
Feedback Mechanisms and Iterative Refinement
The journey continues after the implementation. That's just the beginning. Installing feedback mechanisms where teams can express their experiences, challenges, and suggestions ensures the strategy remains agile and responsive. Regular reviews, perhaps quarterly, where key stakeholders assess the progress against the roadmap, celebrate milestones, and recalibrate the course if needed, are crucial. This iterative approach, where learnings are continuously looped back into the strategy, ensures its long-term relevance and efficacy.
Implementing a Unified Data-Driven CoE Strategy is akin to charting new territories. While the map, in the form of this strategy, provides direction, the journey's success lies in collective will, continuous learning, and the spirit of collaboration. It's about evolving together, with data as the guiding star.
Phase | Duration | Key Activities | Milestones & KPIs | Teams | Tools |
---|---|---|---|---|---|
1 | 2 months | - Conduct in-depth audits of existing data practices, tools, and strategies. - Hold discussions with department heads to identify challenges and expectations. |
- Completion of audit reports. - Documented feedback from all departments. |
- IT/DEV teams - Department heads - External auditors (if required) |
Snowflake & Databricks (for data assessment) |
2 | 1 month | - Organize vision-formulating workshops. - Gather inputs and feedback from all stakeholders. |
- Clear, documented CoE vision statement. | - Executives - Business stakeholders - IT/DEV teams |
Collaborative platforms like Microsoft Teams, Zoom. |
3 | 2 months | - Prioritize components of the CoE strategy. - Develop a phased roadmap with clear milestones and KPIs. |
- Approved roadmap. - Established KPIs for each phase. |
- Strategy team - IT/DEV teams - Business stakeholders |
Project management tools like Jira, Trello. |
4 | 4 months | - Begin integration of Snowflake and Databricks with existing systems. - Train teams on these platforms. |
- Successful system integrations. - Completion of initial training sessions. |
- IT/DEV teams - External consultants (if required) |
Snowflake, Databricks, MuleSoft (for integration). |
5 | 6 months | - Conduct regular training sessions. - Organize knowledge transfer meetups. |
- Measured increase in platform proficiency. - Monthly knowledge-sharing sessions established. |
- All department members - IT/DEV teams - External trainers (if needed) |
E-learning platforms, Snowflake & Databricks training modules. |
6 | Ongoing | - Install feedback mechanisms. - Organize quarterly reviews. - Adapt and refine the strategy based on feedback. |
- Feedback from at least 70% of the organization. - Quarterly review reports. |
- All department members - Executives - Strategy team |
Feedback platforms like Qualtrics, Snowflake & Databricks for analysis. |
This table provides an example of a structured, phased approach to implementing the Unified Data-Driven CoE Strategy. The durations are placeholders and might need adjustments based on the organization's size, complexity, and specific requirements.
Conclusion
In our journey through the intricate realm of a Unified Data-Driven Center of Excellence (CoE) Strategy, we've explored its profound potential for modern organizations. From understanding the individual pillars of data strategy to visualizing the seamless integration of these into a unified vision, we have seen how this approach not only simplifies but amplifies the power of data.
It's worth reiterating that while tools like Snowflake and Databricks provide robust technological backbones, the heart of this strategy lies in its human-centric approach. By bridging gaps, fostering collaboration, and ensuring an organization-wide alignment of objectives, the CoE strategy paves the way for a cohesive, data-driven future.
For executives, this means steering the organization with precision, backed by data-informed insights. For business stakeholders, it translates into understanding customers better, refining marketing approaches, and optimizing operations. For the IT/DEV stakeholders, it represents a harmonized ecosystem where technologies serve clear, aligned business objectives.
In this age, where data is the new oil, organizations that harness its power with a unified, comprehensive approach will undoubtedly lead the pack. They will be the trailblazers, the innovators, the success stories that others aspire to emulate.
As we conclude, I hope you carry forward a renewed appreciation for the transformative power of a unified data strategy and its promise. In unity, there is strength; in strength, there is an unparalleled potential for growth, innovation, and success.
Please leave your comments or DM me directly if you have questions or other inputs I should include.
Member discussion