Why CDPs aren't Personalization Platforms
Okay, back to Martech after my last article on personal finance. I want to touch on personalization in this article. I have written a lot about it in the past, but this one was touched by some recent comments. The topic has been a bane and boon for me over the previous decade. We talk about personalization in various ways in the Martech industry. Mostly to confuse the customer or to make it seem like we have a shiny thing to solve the personalization problem when, in fact, we are liars in most cases.
This week, April 2nd, a notable and respected CTO of a top-tier CDP vendor posted on LinkedIn. He noted this liar issue in the sales process by other CDP vendors as he shared two stories while talking to his customers. He asked whether AI could solve it. Quoted: "Will AI hold vendors accountable for lies told during the sales process?"
While this phenomenon of lying, vaporware, or futures is not new in the software industry, it's especially pronounced in the CDP space. In most cases, CDP vendors lie about what they can realistically do for personalization! It's one of many reasons why CDP projects fail or do not live up to all the hype being promoted and portrayed by various vendors. This doesn't start in sales within these vendors. All Sales teams regurgitate how their Product and Marketing teams have enabled them. Shit flows downhill, as they say - but the entire staff at the vendor creating these fictions should be accountable and seek to change their culture. Speak only to what you can do, not what you want to do. I get it's a crowded field but isn't integrity worth it?
Ironically, while trying to differentiate, Personalization becomes the most prolific of these fictional use cases that CDPs attempt to say they can perform and deliver. Almost none of them can. I will dig into Personalization below and discuss what capabilities a personalization engine should have to fulfill this use case. You can immediately see why a CDP is not a Personalization Platform.
While some CDP platforms can often do what is expected for personalization, for example, Algonomy bought RichRelevance, a pioneer in P13N(Personalization has 13 letters). Adobe is another that has deep P13N capabilities. However, both of these vendors are gluing together a workflow process and explicitly pointing out that there is a different tool or SKU they are selling at each stage of the workflow. But other CDP vendors say they do personalization when, in fact, all they do is facilitate a data pipeline to hold, update, and enrich the profile, forward it on, make it accessible, and inform the personalization platform - they are creating the illusion that they do all the steps in the workflow. A CDP's primary value in P13N is the initial "Profile Resolution" use case. You will see below in the table that many CDPs barely have 1/4 of the capabilities needed for P13N workflow to perform across an Omnichannel environment. The point is that a high % or more of the P13N use cases that CDP vendors sell are BS.
Buyer Beware! Do your due diligence if you need a P13N solution as part of your martech stack or if the CDP vendor is pushing the P13N use case as a huge value add. Dig deep and ask them to prove they can do these things. Make them show you the documentation, like what DynamicYield, RichRelevance, or Adobe would have published on their HELP sites. Most CDPs will not explicitly have technical implementation and API documentation about P13N campaigns and initiatives.
Here's a list of some commonly used terms to help confuse you – the industry even uses the term CDP to replace the Personalization Platform. Dig deep into them to hold the CDP vendor accountable so they can do what they say they can. Maybe they are confused and have a deep personalization platform but selling it now as a CDP. Maybe their marketer is latching on to a market trend and has changed their core value prop. Here are the terms you will see:
AI (Artificial Intelligence) | Refers to machines or software mimicking human intelligence to perform tasks and improve themselves based on the information they collect. Many do not have any AI capabilities. |
ML (Machine Learning) | is a subset of AI that focuses on algorithms that allow software to become more accurate in predicting outcomes without being explicitly programmed to do so. If the CDP vendor has ML staff, they are more akin to having ML models embedded into their platform than actual AI. Algonomy, Amperity, and Adobe have lots of this embedded in their platforms or scattered downstream in their Data apps outside their CDP tools. It does not mean the CDP is a P13N tool if they have ML. |
Deep Learning | is an ML technique that teaches computers to do what comes naturally to humans: learn by example. It's the critical technology behind many advanced AI functionalities, such as speech and image recognition. Personalization tools will have these capabilities—if a CDP vendor does not, they probably can't do P13N well. |
NLP (Natural Language Processing) | is the ability of a computer program to understand human language as it is spoken or written. It is essential for creating chatbots and AI assistants. A few CDP vendors are starting to embed LLM into their backend UIs for the marketer. I talk below about how these tools can feed into the pre-configuration processes and setup for P13N campaigns and tune the P13N engines where the downstream edge ML runs for content delivery. Amperity Algonomy and a few others are moving quickly into these areas. |
Segmentation | divides a customer base into groups of individuals similar in specific marketing-related ways, such as age, gender, interests, and spending habits. This is a CDP core capability. However, you do not need segmentation if you are a P13N vendor because the Deep learning and ML models automate this. For example, Pegasystems and RichRelevance do not need to pre-create or define segments for P13N. They use the whole history of the profile journey to decide on the Content needs for the NBA(next best action, NBO(Next best offer), recommendations, or full/partial page channel content. Audience curation or segmentation is an old, brittle concept for a P13N AI/ML engine. |
Behavioral Targeting | is a technique used by online publishers and advertisers to increase the effectiveness of campaigns through information collected on an individual's web-browsing behavior, such as pages visited or searches made. This capability is foreign to nearly every CDP—if they say they can do BH, they are probably lying. It's a similar adage to the P13N use case. P13N engines do BH as a core feature. Remember CDPs are data-only, and BH and P13N need content plus data. |
Predictive Analytics | is the use of data, statistical algorithms, and machine learning techniques to identify the likelihood of future outcomes based on historical data. Many CDPs have these reports but are not activated into a P13N engine. |
Personalization Engine | Software that uses data and algorithms to provide personalized experiences to users by dynamically inserting, changing, or recommending Content. This is key. They probably do if they say they have this as a separate SKU capability. If the CDP vendor is not talking in these terms but is still promoting P13N, they probably can't do P13N. See my table below and dig deep into the vendors I mentioned above to compare and contrast. |
Recommendation Engine | A capability within a P13N engine that suggests products, services, and information to users based on data analysis. Used extensively in e-commerce sites. This has been the core feature for P13N vendors for a decade or more. It's the default capability and can be deployed subtly across content channels where users would not even know they are being offered a new recommendation. A good P13N engine will have this capability but will give you a UI/UX on both sides of the fence that is subtle and meets 75% of your needs. |
A/B Testing (Split Testing) | is a randomized experiment with two variants, A and B, which are the control and treatment in the controlled experiment. Most P13N engines do not need this as it's already built into the engine - e.g., the engine is continually testing, and your content supply is the key. Suppose you only have 1 variant of offers. In that case, the test is A, so it's up to you and your content developers to feed the engine as much content as possible so it has as many variants. CDPs do not deal in Content; they deal in data management - therefore, ask the CDP vendor how they integrate the content supply chain and product catalog images and inventory into their platforms and how they deliver or render those images and Content to the end user. |
Multivariate Testing | is an extension of A/B testing that tests multiple variables simultaneously to determine the best combination. |
Customer Journey Mapping | is the process of creating a visual story of your customers' interactions with your brand. This narrative helps you understand how a customer experiences your product or service and how they feel along the way. Many CDPs have this tool, but this is not P13N, so don't get lured in on dry land. |
Customer Data Platform (CDP) | is a system that unifies a company's customer data from marketing and other channels to enable customer modeling and optimize the timing and targeting of messages and offers. KEYWORD -- Modeling or optimizing timing -- the CDP is a data pipeline and profile repo, not the actual content deliverer. |
Dynamic Content (or Adaptive Content) | is content that changes based on the viewer's interests or past behavior or depending on conditions set by the marketer. |
Retargeting/ Remarketing | is online advertising targeted to consumers based on their previous Internet actions, where those actions did not result in a sale or conversion. |
Lookalike Audience/ Similar Audience | is a way to reach new people likely to be interested in your business because they're similar to your best existing customers. |
Conversion Rate Optimization (CRO) | is the process of increasing the percentage of users who perform a desired action on a website. Desired actions can include purchasing a product, clicking 'add to cart', signing up for a service, filling out a form, or clicking on a link. |
Engagement Metrics | are analytics that measure the level of engagement of users with a specific piece of Content, often including metrics like time on page, bounce rate, and pages per session. A critical metric for P13N performance. |
Federated Learning | is a machine learning technique that trains an algorithm across multiple decentralized devices or servers holding local data samples, without exchanging them. This newer concept is very exciting at the edge or embedded and incorporates newer AI and deep learning techniques closer to the user. |
Reinforcement Learning | is an area of machine learning concerned with how software agents should act in an environment to maximize cumulative reward. It's not a new technique, but you see newer P13N popups like FLOWRL.ai. I write a bit about a newer role of RL in a previous article and how the newer P13N engines could incorporate a newer model called DPO(Direct Preference Optimization). |
The Imperative for "Always On" Personalization
The "Always On" personalization concept transcends the traditional campaign-based marketing approaches that operate within set timeframes and parameters. The modern consumer journey is a complex web of digital touchpoints, with users seamlessly transitioning across devices, channels, and platforms. This complexity necessitates a marketing system that is perpetually active, dynamically adapting to real-time user data and delivering personalized content experiences that align with each user's current context and needs. CDPs do not have this capability, so I wish they would stop with their P13N use cases, which allude to content delivery abilities. It's flat-out lying to the customer.
Brands that adopt an "Always On" strategy recognize that customer engagement is not a one-time event but a continuous process. Engagement becomes an infinite loop powered by a deep understanding of customer behavior, preferences, and expectations. The "Always On" system is a symphony of software, strategy, and insight, all orchestrated to foster a persistent and personalized relationship with each customer. The CDP is only a small percentage of this workflow, not the entire process.
Architecting for Continuous Engagement
The architecture of an "Always On" personalization system is designed to operate as a living entity, evolving and learning from every interaction. It requires robust foundations in data processing, machine learning, content management, and delivery networks. As I mentioned above in the terms and definitions, most CDPs do not have this P13N AI/ML engine embedded into their core platform. Even Adobe and Algonomy separate this as a composable SKU in other Data apps.
The Data Integration Layer
The data integration layer is central to the system's capabilities. It gathers many data streams essential for painting a complete picture of the customer. Here, data is more than just a resource—it is a beacon that guides the entire personalization journey. Again, as I mentioned in the definitions above, P13N engines all have deep API integration capabilities and, therefore, may only need CDPs for a minor initial load of gold profiles. After that, the CDP would rarely, if at all, ever be needed except to push in new or reload profiles and refresh the entire gold universe.
In this layer, the system synthesizes digital footprints—like web analytics and social interactions—and pulls real-time inventory updates from product catalogs. This integration is crucial for ensuring personalized Content aligns with current product availability, pricing changes, and promotional schedules.
The AI Personalization Engine
The AI Personalization Engine stands at the heart of this system. Beyond its sophisticated pattern recognition and predictive capabilities, the engine is tuned to the rhythm of content consumption and e-commerce dynamics. Content+Data is King with P13N engines. On the other hand, with a CDP, it's only the data.
Most of these engines now leverage deep Learning to map customer interactions against a continually updating product catalog. By doing so, the engine can suggest products that fit the user's profile and are also in stock and optimally priced. The real magic for the P13N engines occurs when they align content themes with product narratives, thus creating a seamless path from brand story to product acquisition.
Furthermore, this engine personalizes the user journey across various dimensions—geographic, demographic, psychographic, and behavioral—ensuring that every piece of Content and product suggestion feels like it was handpicked for the individual user. True 1:1 Marketing Personalization.
The Content and Catalog Synchronization Module
An innovative component within the architecture is the Content and Catalog Synchronization Module. This module ensures that the Content served to users is always in sync with the latest product catalog information. It harmonizes product-related Content, such as descriptions, reviews, and related articles, with current inventory levels, promotional calendars, and pricing strategies.
This synchronization extends to the breadth of the product range, ensuring that recommendations are personalized and practical. Suppose a certain product line is being phased out. In that case, the system will pivot, redirecting user attention toward newer models or alternatives. This enhances the user experience and supports the brand's commercial objectives.
The Content Delivery Network
Personalized Content must reach the user swiftly and reliably, which is the responsibility of the Content Delivery Network (CDN). The CDN sprawls across the edge end-points closest to the end user browser, ensuring that users, irrespective of their location or the device they are using, receive a personalized experience without delay. The idea is to put the content as close to the user as possible to reduce latency and improve the experience. Nearly all P13N platforms have CDNs for content delivery, but almost all CDPs only use CDNs for data collection purposes - this is a Reverse-CDN or pseudo-proxy web server for the CDP. P13N would use it for output and CDPs for input. This is a crucial distinction in how each platform would use a CDN.
A CDN is backed by advanced caching mechanisms that store Content closer to the user, facilitating instantaneous access. It's a dynamic repository that adjusts to the ebb and flow of traffic, product updates, and personalized content demands, ensuring the system's heartbeat is never interrupted.
The User Interface (UI)
In orchestrating the complex interplay between data, AI, and content delivery, the User Interface (UI) provides marketers with a powerful console. Here, data becomes actionable, where insights transform into campaigns, and where the pulse of the personalization engine can be monitored and modulated.
The UI is the nexus through which the synchronization of Content and product catalogs is managed. Marketers can swiftly navigate through product lines, oversee inventory levels, and approve Content that ties into ongoing marketing narratives. They can also harness the UI to sculpt the personalization criteria, define audience segments, and initiate content sequences that resonate with each segment's unique characteristics and current position in the customer journey.
Feedback Loop
Integral to the system's success is the Feedback Loop. This ever-vigilant mechanism ensures that the system is self-correcting and continually improving. The loop fine-tunes the personalization algorithms to enhance accuracy and relevance by analyzing user interactions with content and product recommendations.
Every click, view, and purchase is an input that enriches the system's understanding of user preferences and propels the sophistication of subsequent personalization efforts. This loop is the key to the evolution of the "Always On" experience, ensuring that the system's personalization strategies mature and become more refined with each iteration.
Integrating Content and Commerce
The seamless integration of content and product catalog data sets this "Always On" personalization system apart. It understands that content and commerce are inextricably linked—each narrative journey is a potential pathway to a sale, and each product displays an opportunity for storytelling. By synchronizing the two, the system doesn't just aim to capture attention but seeks to captivate the imagination and encourage commerce in one fluid motion. The AI engine noted above is always-on and listening and delivers across all pathways. The idea is that segments are unnecessary, and the AI engine has access to all the profile attributes and does 1:1 personalization vs. banded segment tiers.
Real-Time Analytics and Adaptive Learning
Within the UI, real-time analytics play a pivotal role, allowing marketers to witness the immediate impact of their content and product strategies. This component of the system is designed to provide a granular view of performance metrics, illuminating patterns that may inform strategic pivots or content refreshes. It's an immersive dashboard where data stories unfold, telling tales of user behaviors, preferences, and even potential churn risks.
Adaptive learning mechanisms within the AI Personalization Engine utilize these analytics to enhance future decision-making. Refer to the CDP checklist article for a list of these reports. These mechanisms interpret the success of content engagement and product interaction, allowing the system to learn from both triumphs and less impactful attempts. The adaptive learning process is crucial for the system's ability to self-optimize, ensuring that personalization becomes more intuitive and effective over time.
Seamless Content-Product Journeys
Marketers can construct seamless journeys that intertwine Content with product discovery. Through the UI, they can map out user pathways that begin with content engagement and organically lead to product pages. The system supports this by providing tools to create dynamic content segments that correspond with specific points in the product catalog, ensuring a frictionless transition from information to transaction.
The integration of Content and commerce is also reflected in how products are showcased within content narratives. The P13N platform often uses advanced tagging and metadata to help embed interactive product highlights within content pieces, allowing users to explore products in a contextual and non-disruptive manner. These product highlights are constantly refreshed to align with the latest catalog data, ensuring accuracy and relevance.
Catalog-Aware Content Personalization
These systems often deploy catalog-aware personalization strategies to ensure personalized content remains relevant and synchronized with the product catalog. These strategies consider factors like seasonal availability, product lifecycle stages, and inventory levels to adapt content recommendations dynamically. Note the table below for a deeper list of the various strategies. Or visit Algonomy's RichRelevance tool and DynamicYield's Resource Help centers for detailed examples.
For instance, if a user shows interest in seasonal items, the system will prioritize content and products relevant to the current season and available inventory. The system anticipates these shifts as seasons change or products end their lifecycle. It adjusts content strategies accordingly, aligning with the user's real-time context and the brand's strategic objectives.
The Marketing Content Repository
Another critical element is the Marketing Content Repository, where all content assets are stored. This repository is not a static vault but a dynamic library that is continuously updated with new assets and pruned of outdated or less effective ones. It serves as the content engine of the system, housing everything from articles and videos to interactive widgets and personalized offer banners. These are separate data apps for products, often called DAMs(Digital Asset Managers). Adobe has one of the best DAMs in the AEM product suite of tools. They are now promoting this as the CONTENT SUPPLY CHAIN. It's a factory that stores and delivers all types of content. P13N vendors usually consume the asset URLs vs. the actual physical asset artifact that the DAM manages and administers. DAM Asset libraries are exposed as public REST URLs - (there is an elaborate workflow behind asset creation, approval and administration), and the P13N engines can easily consume them and serve them as references vs. moving all the physical content. This makes the P13N solution much more integrated, composable, and modular. I do not know one CDP that fully integrates into a DAM solution except Adobe and Algonomy's P13N data apps, which still aren't part of their CDP solution.
The DAM/Asset repository is tightly integrated with the product catalog to enable swift content updates that reflect product offerings or promotional activity changes. This ensures that the content always accurately represents the current state of the product portfolio and marketing initiatives, preserving the integrity and consistency of brand messaging across all channels.
Content-Catalog Matching Engine
The content-catalog matching engine resides at the intersection of content and product data. This newer, sophisticated component uses AI to align Content with the most relevant products in real-time. This is where Adobe is going with the Content Supply chain. There are other vendors in this arena. Adobe is just who I pay attention to. This engine analyzes the content a user engages with to understand their interests and preferences, then matches this with complementary products from the catalog. We will see the merging of the P13N platforms to house multiple engines, similar to my idea on the AI control Plane in one of my previous articles. We will see the converge very quickly so pay close attention to the roadmaps the vendors will share and ask about these tools.
For example, suppose a user reads Content about outdoor activities. In that case, the Matching Engine can recommend relevant outdoor gear from the product catalog, presenting these recommendations within the content experience or as a follow-up, depending on the user's engagement level.
You'll start seeing duality in the P13N engines as AI and AGI become more prevalent. CDPs will be left in the dust if they can't incorporate many of the capabilities that evolved from the P13N engines over the years and leverage this experience. The P13N engines have much better breadth and domain knowledge to grow into these newer engines and add CDP capabilities than the other way around. I suspect the CDP will merge, become a feature of a P13N/Content supply chain factory, and become more invisible in the workflow. The CDP vendors should move towards P13N vs. the other way around.
Infrastructure Scalability and Reliability
The underlying P13N infrastructure is built for scalability and reliability to support the "Always On" mandate. It's an architecture that anticipates peak traffic and scales to accommodate growth. It ensures that the system's uptime meets the highest standards. This means that whether a campaign goes viral or a sale event drives unprecedented traffic, the system remains unflappable, delivering personalized content and product recommendations without missing a beat.
This scalability is mirrored in the P13N system's ability to grow with the brand's expanding product catalog and content portfolio. As new products are introduced and new Content is created, the system seamlessly incorporates these additions into the ongoing personalization strategies without disrupting the user experience.
Personalization Use Cases and Strategies Table
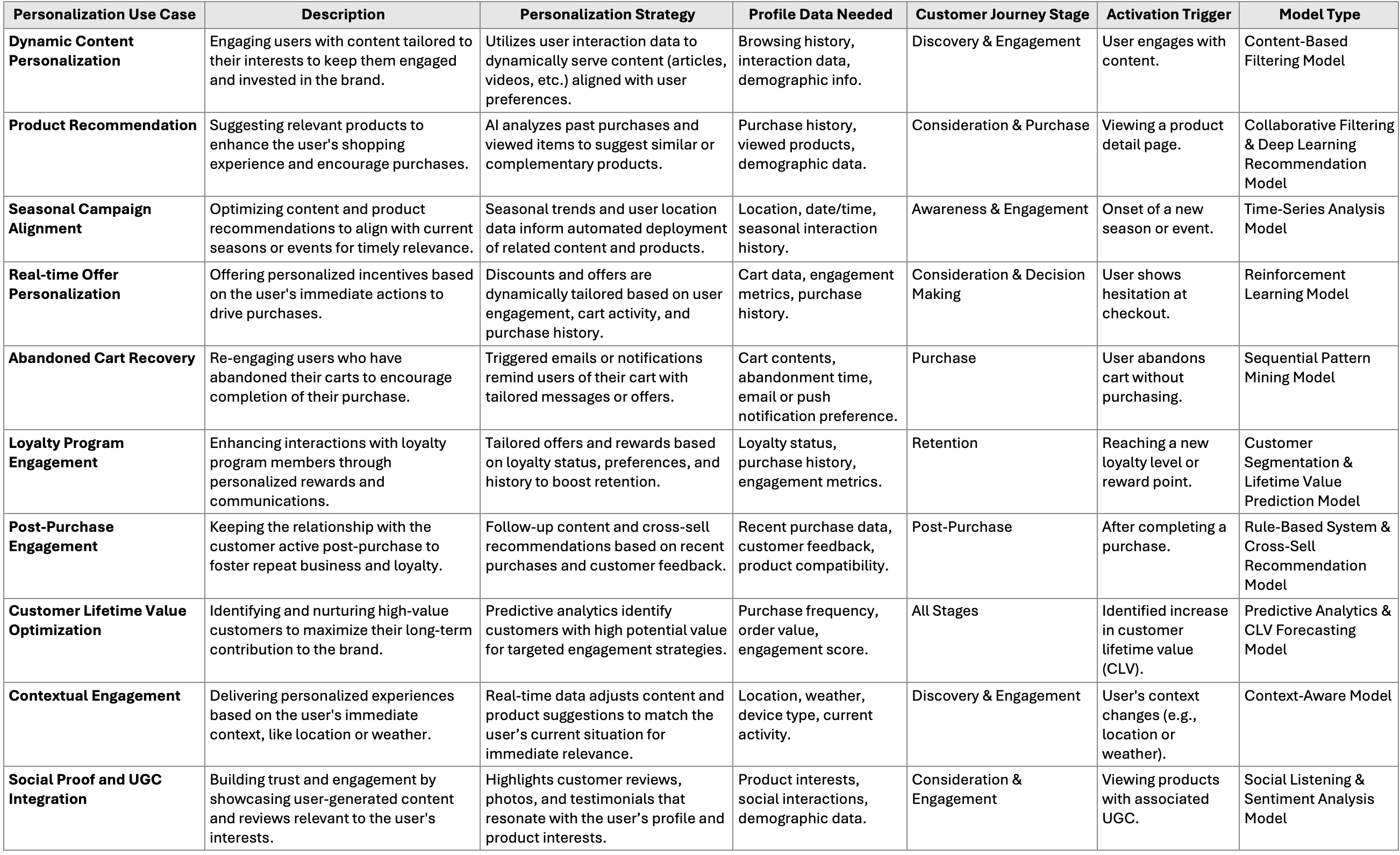
Technical Architecture Ideas
Introduction to Technical Architecture
I want to combine the idea of the "Always On" personalization system with the integration of new LLM AI as an added layer to the UI - updating the older P13N UIs with easier UX flow for the marketer. The newer aspect will still have data analytics and cloud technologies designed to deliver unparalleled personalized user experiences across all brand channels but with better configuration capability in a NLP approach. This architecture could be meticulously crafted to ensure marketers can seamlessly interact with sophisticated AI systems, manage diverse data sources, and deploy personalized Content with precision and agility. I alluded to this in the AI Control Plane article - albeit it was too technical. Still, the idea and premise are where the P13N systems should evolve, thus eliminating the aspect of the CDP as a UI abstraction layer and more as a Data Lakehouse or data management platform. Sitting between these are pipeline, monitoring, and observability.
LLM/NLP AI Systems for Personalization Setup
The integration of large language models (LLM) and natural language processing (NLP) AI systems is now at the forefront of the P13N system's intelligence layer - I previously talked about it as an AI control plane, but that wasn't quite right - I was only conceptualizing at the time. These technologies empower marketers to interact with the system in natural language, simplifying the complex task of setting up personalized scenarios across different channels.
Interaction with LLM/NLP:
- Campaign Initialization: Marketers begin by inputting campaign goals and parameters in conversational language, such as "Increase engagement among 25-34-year-olds interested in outdoor activities in the Northwest region this Spring." The LLM interprets these inputs, translating them into detailed campaign specifications. It creates a campaign definition but not the decision models for the content. That happens downstream with the real-time AI engine and ensemble models that decide what content is for what profile based on the campaign definition. Notice this is not a segment per se but more of a meta-segment, including the campaign brief and definition.
- Data Querying and Profile Setup: (Optional) The system utilizes an abstracted cross-cloud querying mechanism connected to a Customer Data Platform (CDP) or a data lakehouse like Snowflake - preferably, these are the same, or the P13N could be fully integrated. I wanted to give the CDP the benefit of the doubt - perhaps this is more of a Reverse ETL-type tool. It should be invisible or highly abstracted for the end user. This part depends more on the profile master and where the real-time updates and events occur without causing latency. Here, marketers can specify the profiles to target or exclude using a simplified query interface powered by TrinoSQL-like technology. In P13N parlance, it's called Boosting or Restricting, AKA business rules. This step ensures the campaign draws on comprehensive, up-to-date customer data without requiring deep technical expertise.
- Personalization Strategy Configuration: (Optional) Based on the initial prompts, the LLM could suggest several personalization strategies and models suitable for the campaign's goals. These could be similar to the strategies in the table above or this article I wrote several months ago. Marketers can review, modify, or approve these suggestions directly within the UI, tailoring each strategy to their needs.
Data Integration and Management
The backbone of the "Always On" system is its robust data integration and management capability. The system creates a holistic view of each customer by harnessing data initially from the CDP as a single load or refresh and keeping its profile store current from social media interactions, transactional databases, and external data sources as needed. Depending on the need, latency, and type of content, the profile could be a metaID system or a fully stored profile.
- Real-Time Data Syncing: Continuous syncing with the CDP ensures that customer profiles are always current, reflecting the latest interactions, transactions, and behaviors. While I have downplayed this as a core need, the CDP could do this, but I do not think it should evolve in this direction. You only need a data platform like a Lakehouse, or the P13N system can have its own profile store with CDP capability. In my opinion, this middle-brokeresque system is redundant and unneeded as long as the Lakehouse layer or the P13N profile store can incorporate the other part-time features the CDP provides.
- Segmentation and Enrichment: A good P13N engine doesn't need segmentation. However, marketers will always ask for this feature. So, the best way to provide it is as an advanced META-segmentation tool. This tool allows marketers to define target groups based on demographic, behavioral, and psychographic data, as mentioned above, to define the campaign they want to promote using LLM in an NLP fashion. The system could also automatically enrich these segments with predictive insights, aka scores, enhancing campaign precision, but normally, this happens downstream in the P13N delivery engine. A really good mixed LLM + AI P13N engine with a model stack doesn't need segmentation as it targets 1:1 at all times.
AI Engines and Embedded Models at the Edge
Personalization decisions are executed at the edge of the network, closest to the user, ensuring real-time content delivery and interaction.
- Edge AI Deployment: Embedded AI models at the edge analyze incoming user data in real-time, deciding which content variant or product recommendation to serve based on the user's current context and historical data.
- Content Delivery Optimization: The system dynamically selects the optimal content delivery path, adjusting for network conditions, device capabilities, and user preferences to ensure a seamless experience.
Implementation Journey and Deployment by Marketers
Marketers are central to bringing "Always On" personalization to life. Let's walk through their UI journey again. Using the system's UI, they get a more intuitive and empowering UX flow, enabling them to launch sophisticated campaigns quickly. The idea is to reduce and abstract UI/UX for them, removing the CDP flows for the marketer, lowering P13N engine configs, and converting commands to NLP prompts.
- Campaign Setup: Using the LLM/NLP interface, marketers set up campaigns by describing their goals and target audience in plain language. The system then guides them through selecting and configuring the appropriate personalization strategies and AI models.
- Data Querying and Profiling: Marketers refine their audience meta-segments through a user-friendly interface, leveraging real-time data insights to tailor campaigns to specific user needs and preferences.
- Content and Product Catalog Integration: Marketers synchronize Content and product catalogs, ensuring that all personalized recommendations are up-to-date and relevant.
- Real-time Monitoring and Adjustment: The system provides real-time analytics and performance metrics, enabling marketers to adjust strategies on-the-fly to optimize campaign outcomes.
Future Trends in Personalization
To ensure the "Always On" personalization system remains at the forefront of digital marketing technology, integrating emerging trends and advancements in artificial intelligence, especially in areas like deep Learning, Large Language Models (LLMs), and Reinforcement Learning from Human Feedback (RLHF), is crucial, DPO(Direct Preference Optimization) may be the next evolution. These technologies are rapidly evolving, edging closer to the capabilities associated with Artificial General Intelligence (AGI), and promise to enhance the sophistication and effectiveness of personalization strategies significantly.
- Deep Learning Evolution: Many P13N engines already incorporate this into the system. They will increasingly utilize advanced deep-learning techniques to process and interpret the vast amounts of unstructured data generated by user interactions. This includes a more nuanced understanding of images, videos, and complex user behaviors, allowing for richer, more contextually aware personalization. Techniques like convolutional neural networks (CNNs) and recurrent neural networks (RNNs) will evolve to analyze temporal patterns and sequences in user data, offering predictions with unprecedented accuracy.
- Expansion of LLM Capabilities: LLMs, such as GPT and its successors, are expected to become more integral to personalization systems. Some CDPs are already adding the capability, but I wonder if they can incorporate P13N as the layer adjacent to these tools. Based on what I have seen with CDP vendors, I highly doubt it. They do not have the domain expertise to pull it off. Most of the capabilities already released are garbage. LLM/GPT's ability to generate human-like text and understand user queries with high precision will enable more natural and engaging interactions. Future developments will likely see LLMs offering more customized content creation, automatically generating personalized articles, product descriptions, and marketing messages that resonate deeply with individual users.
- Reinforcement Learning from Human Feedback (RLHF): This technique combines the power of reinforcement learning (RL) with human feedback to refine AI outputs. In the context of "Always On" personalization, RLHF can be used to continuously improve the relevance and timing of personalized Content and recommendations. Personalization strategies will become increasingly aligned with user preferences and behaviors by incorporating user feedback directly into the learning loop, enhancing user satisfaction and engagement. I am a big fan of the next update to RL with DPO, which I have mentioned multiple times in this article above. This model will significantly impact streamlining or eliminating the heavier models already deployed in the P13N engines.
- Towards Artificial General Intelligence (AGI): As we move closer to the realization of AGI, personalization systems will begin to exhibit more advanced understanding and predictive capabilities, approaching human-like intuition in marketing decision-making. AGI would enable the system to understand complex user needs, predict future behaviors, and autonomously develop innovative personalization strategies that adapt to changing market dynamics and consumer trends.
- Retrieval-Augmented Generation (RAG): This approach combines the generative capabilities of models like GPT with the information retrieval abilities of models akin to BERT (Bidirectional Encoder Representations from Transformers). For "Always On" personalization, RAG allows for the dynamic generation of Content that is both deeply personalized and informed by the latest, most relevant information. This means marketing messages can be tailored not just to the user's profile but also to current events, trending topics, and the latest product information, ensuring maximum relevance and engagement.
- Ethical AI and Transparency: As AI models become more powerful, ensuring their ethical use and transparency in how personalization decisions are made will become paramount. Advances in explainable AI (XAI) will make it possible to understand and communicate the reasoning behind AI-generated personalization strategies, fostering trust between brands and consumers.
- Privacy-First Personalization: In the era of increasing data privacy concerns and regulations, advances in machine learning, especially federated Learning and differential privacy, will enable the "Always On" system to deliver highly personalized experiences without compromising user privacy. These technologies allow for the training of AI models on decentralized data, minimizing the risk of data breaches and ensuring compliance with privacy laws.
By integrating these trends, the "Always On" personalization system will keep pace with the rapidly evolving digital landscape and redefine the boundaries of what's possible in creating deeply personalized, engaging, and respectful user experiences. CDPs and P13N should evolve and become easier for the end user to use. The current UI/UX abstractions are impossible. They need to collapse and simplify while the backends maintain their composable separations so anyone can build UI/UX that suits them.
I hope you enjoyed this article and if you would like to talk more or refer me to others for job consideration please DM me.
Member discussion